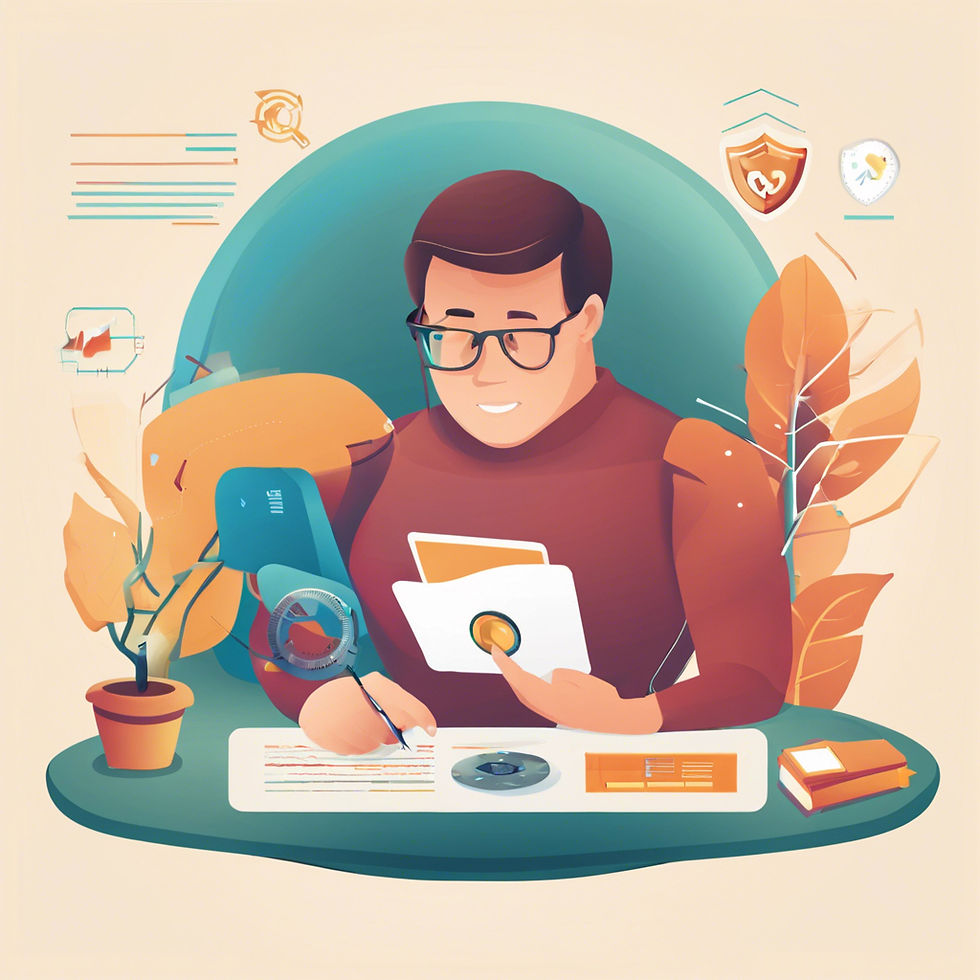
As health research increasingly leverages artificial intelligence (AI) and digital technologies, the importance of data privacy and security has never been more critical. The vast amount of sensitive information collected, analyzed, and stored presents both tremendous opportunities and significant risks. In the age of AI, where data is the fuel that powers innovation, ensuring that this data is handled securely and ethically is paramount. This blog post explores the challenges of data privacy and data breaches in health research and offers strategies for prevention.
The Rising Threat of Data Breaches in Health Research
Health research often involves the collection of highly sensitive personal information, including medical histories, genetic data, and other private details. The integration of AI into this research amplifies the risks, as AI systems require large datasets to function effectively. Unfortunately, this makes health research a prime target for cyberattacks and data breaches.
A data breach in health research can have devastating consequences, not only for the individuals whose privacy is compromised but also for the integrity of the research itself. Breached data can lead to identity theft, discrimination, and loss of trust among participants. Moreover, the research community could face legal ramifications, loss of funding, and reputational damage.
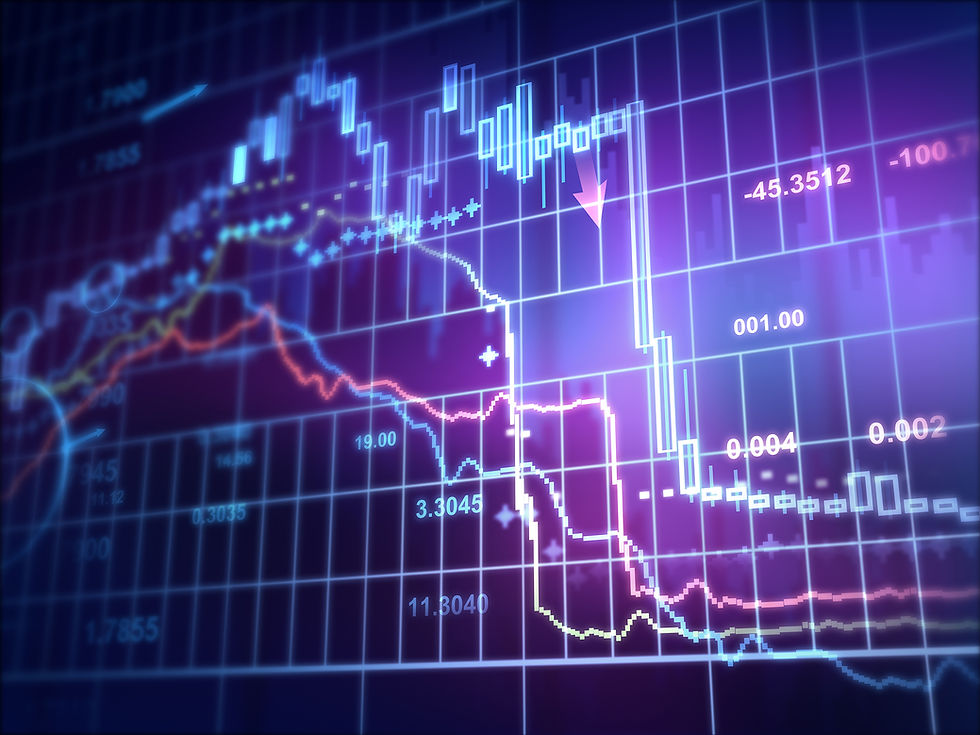
Key Challenges in Protecting Data Privacy
Data Volume and Complexity:
AI-driven health research often involves processing massive datasets, which can be challenging to secure. The sheer volume of data increases the potential attack surfaces, making it easier for malicious actors to find vulnerabilities.
Data Sharing and Collaboration:
Collaborative research across institutions and borders is common in health research. While this fosters innovation, it also complicates data security, as data is transferred between different systems and jurisdictions with varying levels of protection.
Ethical Use of AI:
AI algorithms can inadvertently reinforce biases or make decisions based on sensitive attributes like race, gender, or socioeconomic status. Ensuring that AI systems respect privacy while also making ethically sound decisions is a complex challenge.
Regulatory Compliance:
Health research must comply with strict regulations such as the Health Insurance Portability and Accountability Act (HIPAA) in the United States or the General Data Protection Regulation (GDPR) in the European Union. Navigating these regulations while conducting innovative research can be difficult, especially when AI systems are involved.
Strategies for Preventing Data Breaches in Health Research
Implement Strong Encryption Protocols:
Encrypting data both at rest and in transit is one of the most effective ways to protect sensitive information. Even if data is intercepted, encryption ensures that it remains inaccessible without the appropriate decryption keys.
Adopt Privacy-by-Design Principles:
Privacy-by-design involves integrating privacy protection into the development of AI systems and research protocols from the outset. This means considering privacy implications during the design phase and ensuring that all data handling processes prioritize confidentiality and security.
Regular Security Audits and Penetration Testing:
Conducting regular security audits and penetration tests can help identify vulnerabilities in your systems before they can be exploited. These assessments should be performed by cybersecurity professionals who can provide insights into potential weaknesses and offer recommendations for improvement.
Limit Data Access:
Implementing strict access controls ensures that only authorized personnel can access sensitive data. This can be achieved through role-based access controls (RBAC) and multi-factor authentication (MFA), which add layers of security to prevent unauthorized access.
Anonymization and De-Identification:
Whenever possible, data should be anonymized or de-identified to protect the privacy of research participants. This process involves removing or obfuscating personal identifiers, making it difficult to trace data back to individuals while still allowing for valuable research insights.
Compliance with Regulations:
Staying informed about relevant data protection regulations and ensuring compliance is critical. This includes not only adhering to laws like HIPAA and GDPR but also staying updated on any new regulations that may affect AI-driven research.
Educating Researchers and Staff:
Data privacy and security should be a priority for everyone involved in the research process. Regular training sessions on best practices for data handling, recognizing phishing attempts, and responding to potential breaches are essential for maintaining a secure environment.
Ethical AI Development:
Developing AI systems that are transparent, explainable, and free from biases is crucial. This involves rigorous testing of AI models to ensure they do not inadvertently compromise privacy or make unethical decisions based on sensitive data attributes.
Conclusion
As AI continues to transform health research, the stakes for data privacy and security are higher than ever. Preventing data breaches in this context requires a comprehensive approach that combines strong technical measures with ethical considerations and regulatory compliance. By implementing these strategies, researchers can protect the privacy of their participants, uphold the integrity of their work, and contribute to the responsible advancement of health research in the age of AI.
Comments